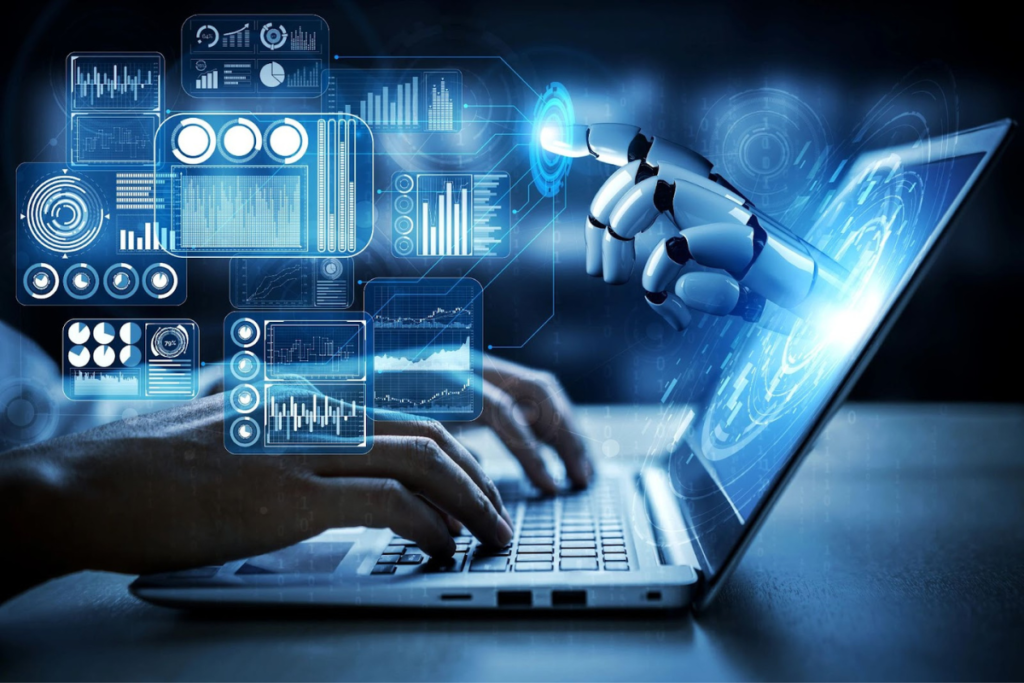
In recent years, Artificial Intelligence (AI) and Machine Learning (ML) technologies have become increasingly popular and are transforming industries of all sizes. According to a McKinsey report, businesses that utilize AI technologies will double their cash flow by 2030. Conversely, companies that do not deploy AI will see a 20% reduction in their cash flow. However, poor quality data hinders the ability of companies to utilize AI and ML effectively. A PwC survey conducted in 2019 found that only 15% of companies had access to high-quality data to achieve their business goals.
Poor data quality is the main hindrance to businesses in obtaining high-quality AI-powered analytics. According to a survey by Refinitiv, 66% of respondents said that poor quality data impairs their ability to deploy and adopt AI effectively. The top three challenges of working with ML and AI technologies were “accurate information about the coverage, history, and population of the data,” “identification of incomplete or corrupt records,” and “cleaning and normalization of the data.”
So why is data quality important in AI and ML applications? Firstly, the output heavily depends on the input – garbage in, garbage out. If the data sets are full of errors or skewed, the result will also be inaccurate. Poor data quality leads to the inability of AI models to function correctly. Secondly, not all AI systems are created equal. Some AI models can handle both quantitative and qualitative datasets, while others can handle only one type. It is essential to select the right data type for the appropriate model to get the expected output.
Thirdly, quality is preferable to quantity. It is commonly believed that AI systems need to ingest a lot of data to learn from it. However, high-quality datasets that are shorter in nature can provide relevant and robust output. Fourthly, a good dataset has specific characteristics that one must look for when analyzing it. These include completeness, comprehensiveness, consistency, accuracy, and uniqueness.
To ensure that data quality is high, one must ensure that the data source is trustworthy. There are many ways to ensure that the data quality is high, such as data profiling, evaluating data quality, monitoring and evaluating data quality, and data preparation. Data profiling offers insight into the distribution of values, maximum, minimum, and average values, and outliers. Evaluating data quality involves validating any dataset with a central library of pre-built data quality rules. Monitoring and evaluating data quality involves narrowing down to specific issues of an attribute and deciding whether to use that attribute or not. Finally, data preparation involves tweaking data to prepare it for AI modeling.
Takeaway
In summary, the role of data quality cannot be overstated in the realm of AI and ML applications. It serves as the foundation upon which these technologies build insights and recommendations. If the quality of data fed to these algorithms is subpar, it can lead to flawed and unreliable results. Hence, it is essential for businesses to prioritize data quality management to harness the full potential of AI-powered analytics.
The benefits of high-quality data are manifold – it can reduce the need for large datasets, leading to more efficient and cost-effective data processing. It can also help companies gain a competitive edge by providing accurate and actionable insights that drive better decision-making. Additionally, good data quality management practices can help companies meet regulatory compliance requirements and build trust with customers.
Moreover, it is worth noting that data quality is not a one-time task but an ongoing process. As businesses continue to evolve and generate more data, they need to continually monitor and improve the quality of their data. By investing in data quality management tools and processes, companies can mitigate the risk of poor data quality, eliminate bottlenecks in the AI and ML workflows, and unlock new opportunities for innovation and growth.
In conclusion, data quality is a foundational pillar for successful AI and ML applications. By prioritizing data quality management, companies can gain significant benefits, including better accuracy, efficiency, compliance, and innovation. Therefore, it is crucial for organizations to make data quality management a top priority to reap the full benefits of AI and ML technologies.